Machine Learning for Mechanics
The field of mechanics has long relied on simple theoretical material models, which, while valuable for understanding material behavior, represent a simplification of reality. Currently, significant progress is being made in the development of machine-learning techniques capable of modeling complex systems. The intersection of machine learning and material modeling holds immense promise for the future, provided that machine learning tools respect the constraints imposed by nature. However, the precise methods for achieving this integration remain a critical open question.
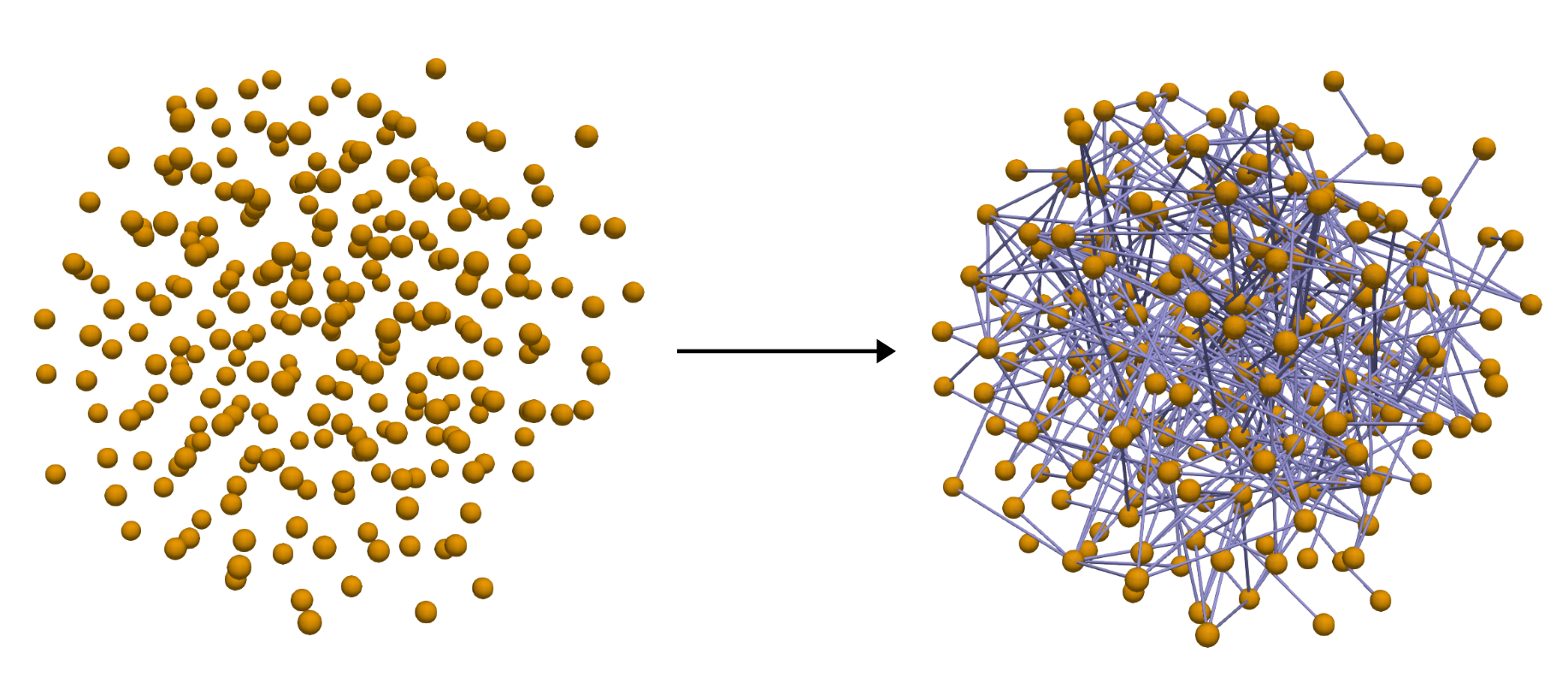
In our work, we aim to develop cutting-edge machine-learning methods that account for material complexities such as heterogeneities. By using graph neural networks, we reveal the underlying material model without relying on prior domain knowledge. This includes discovering the interaction between discrete particles in granular materials solely through observation of their movements, as well as learning the stress-strain constitutive material laws of solids. These methodologies enable a systematic approach to determining material models, advancing our understanding of material behavior, and facilitating the development of accurate predictive tools.